Of all things, which is the most likely to end life on Earth as we know it: a meteorite strike, extreme climate change, a pandemic, a solar flare?
A new statistical method could help scientists more accurately analyze worst (or best) case scenarios by teasing out information about events that are rare, but highly consequential. The method could help everyone from investors to government officials and insurance companies make informed decisions on potential dangers where data is sparse.
“Though they are by definition rare, such events do occur, and they matter,” said mathematical biologist Joel E. Cohen, a coauthor of the research. “We hope this is a useful set of tools to understand and calculate these risks better.” Cohen is a professor at Rockefeller University and Columbia University’s Earth Institute, and currently a visiting scholar at the University of Chicago. The research was just published in the Proceedings of the National Academy of Sciences.
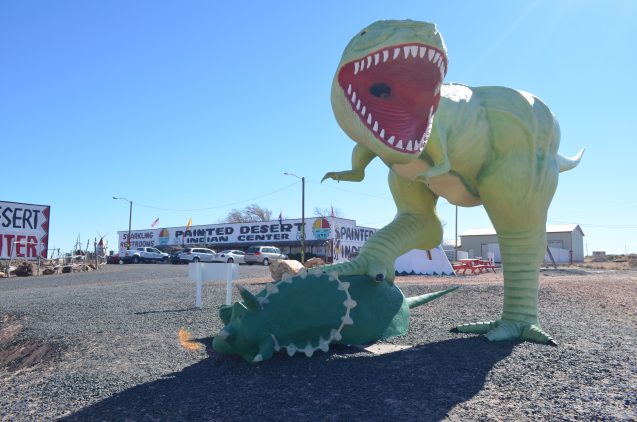
Statistics is the science of using limited data to learn about the world. Its questions range from “What is the best time to spray pesticides on a field of crops?” to “How likely is it that a global pandemic will shut down large swaths of public life?” A century old, the statistical theory of rare-but-extreme events is a relatively new field, and scientists are still cataloguing the best ways to crunch different kinds of data. Calculation methods can significantly affect conclusions, so researchers have to carefully tune their approaches to the data.
Two powerful tools in statistics are the average and the variance. Most people are familiar with the average: If one student scores 80 on a test and another scores 82, their average is 81. Variance, on the other hand, measures how widely spread out those scores are. You would get the same average of 81 if one student scored 62 and the other 100, but the classroom implications would be very different.
In most situations, both the average and the variance are finite numbers. But things get stranger when you look at disastrous events that are extremely rare. In most years, there is no gigantic burst of activity from the Sun’s surface big enough to fry all of Earth’s electronics—but one could be remotely possible, and if it happened this year, the results would be catastrophic. Similarly, the vast majority of business startups fizzle out, but occasionally a Google or Facebook comes along.
“There’s a category where large events happen very rarely, but often enough to drive the average and/or the variance towards infinity,” said Cohen.
These situations require their own special tools. And understanding their risk (known in statistics parlance as events with “heavy-tailed distribution”) is important for many people. Government officials need to know how much effort and money they can reasonably invest in disaster preparation; investors want to know how to maximize returns and still take into consideration highly unlikely scenarios.
Cohen and his colleagues looked at a mathematical model recently used to calculate risk. This model splits the variance in the middle and calculates the variance both above and below the average. This is designed to give more information about both downside risks and upside risks. For example, a new tech company may be found to be much more likely to fail (that is, to wind up below the average) than to succeed (wind up above the average). This is something a potential investor might want to know. However, this method had not been examined for distributions of low-probability, very high-impact events with infinite mean and variance.
Running tests, the scientists found that standard ways to work with these numbers, called semi-variances, don’t yield much information. But they found other ways that did. For example, they could extract useful information by calculating the ratio of the log of the average to the log of the semi-variance. “Without the logs, you get less useful information,” said Cohen. “But with the logs, the limiting behavior for large samples of data gives you information about the shape of the underlying distribution, which is very useful.”
“We think there are practical applications for financial mathematics, for agricultural economics, and potentially even epidemics. But since it’s so new, we’re not even sure what the most useful areas might be,” Cohen said. “We just opened up this world.”
The researchers do not claim to know quite yet what is most likely to end life on Earth.
The other authors of the study are Mark Brown of Columbia University; Chuan-Fa Tang of the University of Texas Dallas; and Sheung Chi Phillip Yam of the Chinese University of Hong Kong.
Adapted from a press release by the University of Chicago.