A novel glacier model has been developed which can simulate ice dynamics and ice interaction with the climate up to a thousand times faster than previous models. This model can be used to predict the evolution of glaciers and ice sheets under different scenarios. Since meltwater from glaciers and ice sheets is a major component of sea level rise, models like this are a valuable tool to assess their potential future contribution.
The new model uses a machine learning approach which makes glacier modeling much quicker whilst maintaining high levels of fidelity (the degree to which a simulation or model accurately reproduces the object or process it is designed to represent). As a result, more model simulations with different inputs and assumptions can be conducted, investigating a wider range of questions.
The state of the art Instructed Glacier Model is highly efficient compared to well-established simulation tools. It implements an artificial neural network, which is a computer system that mimics the neural networks found in our brains. They ‘trained’ the neural network by inputting data from ice sheet models so that it could emulate ice dynamics. This training process is called machine learning, and it is considered part of the field of artificial intelligence. Modeling methods prior to AI required a great deal of human input, supervision and decision making, whereas with machine learning, the computer system navigates the human process of updating the model on its own.
The lead developer, Guillaume Jouvet, a senior researcher at the University of Zurich, explained that “[there is] a new trend for machine learning to learn from data generated by physical models.” Physics-based models (also termed physical models) have long been used to understand the physical processes occurring in the Earth system, without relying on any artificial intelligence.
Physical modeling of ice sheets and glaciers at high spatial resolutions is an enormous challenge even today. Over the past two decades, exceptional efforts have been made to develop models to simulate ice flow and its associated physical processes, as well as its interaction with the climate. Adding complexity to models increases the computational cost of the simulation, so most models often use approximations to the Stokes equations, which most faithfully describe ice flow, entailing a compromise between accuracy and computational cost. Jouvet describes that the main motivation behind transitioning to machine learning is “in a way, you are shortcutting your physical modeling, making the gain computationally way cheaper.”
GlacierHub spoke with Laura Sandoval, of the University of Colorado, Boulder, who led a review into artificial intelligence in the geosciences field. “In the past decade the AI [and] machine learning activities have increased tremendously in the field of geosciences, [but] most AI efforts in geoscientific research groups are still at the infancy stage,” she stated. “Currently, researchers are actively exploring many AI models and prototyping solutions for the challenging problems within their domains.” However, in comparison to the traditional physics-based models, there have been no big breakthroughs in AI and machine learning products yet. Sandoval added “the implementation of AI is still underway.”
The Instructed Glacier Model substitutes the most computationally demanding model component by using a neural network trained from large datasets. Taking advantage of the large amount of modeling data available to train the neural network delivers high fidelity solutions at much lower computational cost. It can predict ice flow from given variables and simplified processes to be used in global glacier modeling as well as researching past glaciated environments.
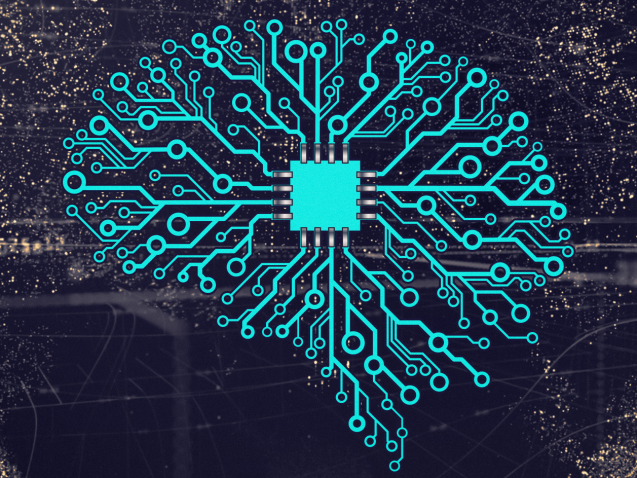
“The most expensive part was computing the dynamics because it involved heavy physics, [but] machine learning accelerated this part of the model. The result is that we can model the glacier to the same accuracy much quicker than before. We can use this to explore many more parameters and [conduct] more refined simulations,” said Jouvet. The research on the model took Jouvet and his team over a year. He added that “I had to learn this new technique — all the tools I’m using are really new.”
The researchers are pleased that they were able to have the machine learning up and running and Jouvet will now look forward to using his model to reconstruct the evolution of glaciers in the Alps over the last glacial cycle of 100,000 years. “The gain for this approach is you speed up the modeling so you can afford to do long timescales. [Where] traditional models may take several weeks, it can now take an hour.”
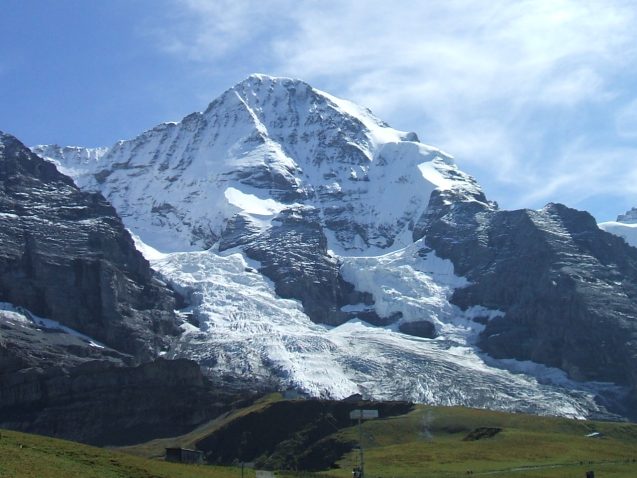
Implementation of AI and machine learning does not come without its challenges and skepticism, similar to that seen in high-profile cases in biology and engineering. Sandoval explains “Ethics is truly one of the major concerns. However, since we are still at an early prototyping stage, the current main arguments against AI are uncertainty, explainability and reproducibility.” Ethical issues surrounding AI include the loss of human jobs, the unequal distribution of wealth created by AI machines, the security of AI data and the capacity for malicious intent. As implementation of AI increases, more concerns are emerging, such as the environmental issues of using large amounts of energy to run computer models. Similar arguments have been widely seen against other cyber services like cryptocurrency and electronic trading.
Scientists have been studying big questions about our climate and Earth system for many years and have accumulated a large amount of data which will be used to train AI models. “Given the recent huge investments in AI from both the public and private sectors, we expect to see that the relevant application on data-centric AI research in geosciences will bloom in the next few years,” says Sandoval.
Despite the transition, not every geoscientific problem can be solved by AI, and some questions are not well suited to classic machine learning techniques. “Some Earth phenomena are extreme events and their patterns cannot be learnt from historical data. Finding a suitable question is the key first step to develop a successful AI application,” Sandoval concludes.
The novel Instructed Glacier Model is a successful example of how new techniques for glacier modeling may replace the traditionally known physics-based approaches. Many uncertainties surrounding artificial intelligence still remain and whether large-scale progress in the field will be seen is a question of the coming decade. For now, both old and new techniques will be implemented in order to provide answers to some of our greatest questions regarding ice sheets and glaciers.