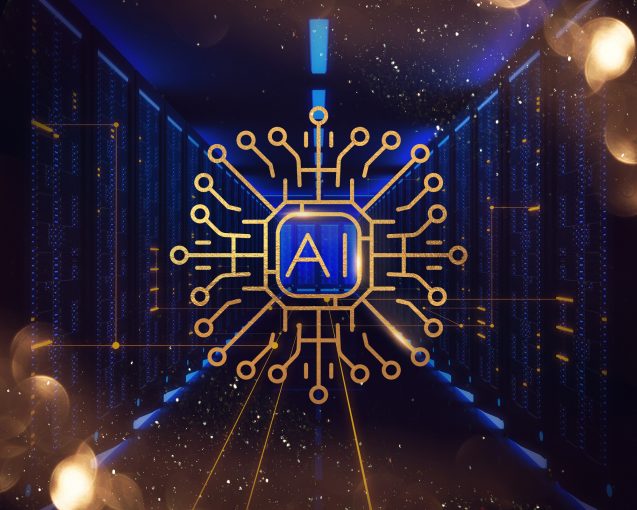
These days, the buzz is all about artificial intelligence (AI), computer systems that can sense their environment, think, learn, and act in response to their programmed objectives. Because of AI’s capabilities, there are many ways it can help combat climate change, but will its potential to aid decarbonization and adaptation outweigh the enormous amounts of energy it consumes? Or will AI’s growing carbon footprint put our climate goals out of reach?
The artificial intelligence revolution
Earlier this year, several large language models—revolutionary types of AI trained on huge amounts of text that can generate human sounding text—were launched. The first large language model appeared in the 1950s, but today’s models are vastly more sophisticated. The most popular new models are Microsoft’s AI-powered Bing search engine, Google’s Bard, and OpenAI’s GPT-4.
When I asked Bard why large language models are revolutionary, it answered that it is “because they can perform a wide range of tasks that were previously thought to be impossible for computers. They can generate creative text, translate languages, and answer questions in an informative way.” Large language models are a type of generative AI, because the models can generate new combinations of text based on the examples and information they have previously seen.
How do large language models work?
The goal of a large language model is to guess what comes next in a body of text. To achieve this, it first must be trained. Training involves exposing the model to huge amounts of data (possibly hundreds of billions of words) which can come from the internet, books, articles, social media, and specialized datasets. The training process can take weeks or months. Over time, the model figures out how to how to weigh different features of the data to accomplish the task it is given. At first a model’s guesses are random, but as training progresses, the model identifies more and more patterns and relationships in the data. The internal settings that it learns from the data are called parameters; they represent the relationships between different words and are used to make predictions. The model’s performance is refined through tuning, adjusting the values for the parameters to find out which ones result in the most accurate and relevant outcomes.
Each generation of large language models has many more parameters than the previous one; the more parameters, the more accurate and flexible they can be. In 2018, a large language model had 100 million parameters. GPT-2, launched in 2019, had 1.5 billion parameters; GPT-3 at 100 times larger, had 175 billion parameters; no one knows how large GPT-4 is. Google’s PaLM large language model, which is much more powerful than Bard, had 540 billion parameters.
To process and analyze the vast amounts of data, large language models need tens of thousands of advanced high-performance chips for training and, once trained, for making predictions about new data and responding to queries.
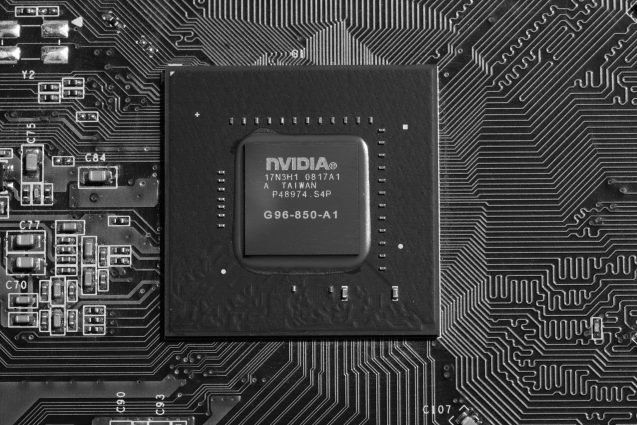
Graphics processing units (GPUs), specialized electronic circuits, are typically used because they can execute many calculations or processes simultaneously; they also consume more power than many other kinds of chips.
AI mostly takes place in the cloud—servers, databases, and software that are accessible over the internet via remote data centers. The cloud can store the vast amounts of data AI needs for trainings and provide a platform to deploy the trained AI models.
How AI can help combat climate change
Because of its ability to analyze enormous amounts of data, artificial intelligence can help mitigate climate change and enable societies to adapt to its challenges.
AI can be used to analyze the many complex and evolving variables of the climate system to improve climate models, narrow the uncertainties that still exist, and make better predictions. This will help businesses and communities anticipate where disruptions due to climate change might occur and better prepare for or adapt to them. Columbia University’s new center, Learning the Earth with Artificial Intelligence and Physics (LEAP) will develop next-generation AI-based climate models, and train students in the field.
AI can help develop materials that are lighter and stronger, making wind turbines or aircraft lighter, which means they consume less energy. It can design new materials that use less resources, enhance battery storage, or improve carbon capture. AI can manage electricity from a variety of renewable energy sources, monitor energy consumption, and identify opportunities for increased efficiency in smart grids, power plants, supply chains, and manufacturing.
AI systems can detect and predict methane leaks from pipelines. They can monitor floods, deforestation, and illegal fishing in almost real time. They can make agriculture more sustainable by analyzing images of crops to determine where there might be nutrition, pest, or disease problems. AI robots have been used to collect data in the Arctic when it is too cold for humans or conduct research in the oceans. AI systems can even green themselves by finding ways to make data centers more energy efficient. Google uses artificial intelligence to predict how different combinations of actions affect energy consumption in its data centers and then implements the ones that best reduce energy consumption while maintaining safety.
These are just a few examples of what AI can do to help address climate change.
How much energy does AI consume?
Today data centers run 24/7 and most derive their energy from fossil fuels, although there are increasing efforts to use renewable energy resources. Because of the energy the world’s data centers consume, they account for 2.5 to 3.7 percent of global greenhouse gas emissions, exceeding even those of the aviation industry.
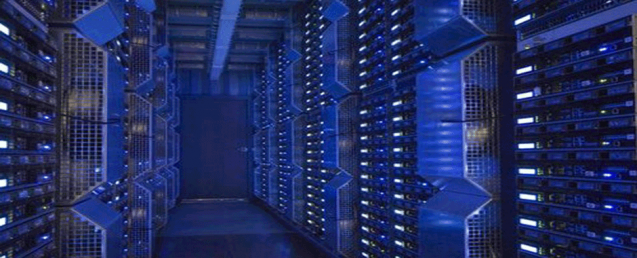
Most of a data center’s energy is used to operate processors and chips. Like other computer systems, AI systems process information using zeros and ones. Every time a bit—the smallest amount of data computers can process—changes its state between one and zero, it consumes a small amount of electricity and generates heat. Because servers must be kept cool to function, around 40 percent of the electricity data centers use goes towards massive air conditioners. Without them, servers would overheat and fail.
In 2021, global data center electricity use was about 0.9 to 1.3 percent of global electricity demand. One study estimated it could increase to 1.86 percent by 2030. As the capabilities and complexity of AI models rapidly increase over the next few years, their processing and energy consumption needs will too. One research company predicted that by 2028, there will be a four-fold improvement in computing performance, and a 50-fold increase in processing workloads due to increased use, more demanding queries, and more sophisticated models with many more parameters. It’s estimated that the energy consumption of data centers on the European continent will grow 28 percent by 2030.
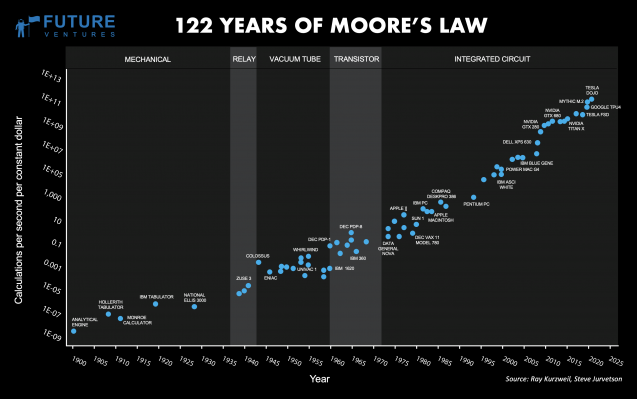
With AI already being integrated into search engines like Bing and Bard, more computing power is needed to train and run models. Experts say this could increase the computing power needed—as well as the energy used—by up to five times per search. Moreover, AI models need to be continually retrained to keep up to date with current information.
Training
In 2019, University of Massachusetts Amherst researchers trained several large language models and found that training a single AI model can emit over 626,000 pounds of CO2, equivalent to the emissions of five cars over their lifetimes.
A more recent study reported that training GPT-3 with 175 billion parameters consumed 1287 MWh of electricity, and resulted in carbon emissions of 502 metric tons of carbon, equivalent to driving 112 gasoline powered cars for a year.
Inference
Once models are deployed, inference—the mode where the AI makes predictions about new data and responds to queries—may consume even more energy than training. Google estimated that of the energy used in AI for training and inference, 60 percent goes towards inference, and 40 percent for training. GPT-3’s daily carbon footprint was been estimated to be equivalent to 50 pounds of CO2 or 8.4 tons of CO2 in a year.
Inference energy consumption is high because while training is usually done multiple times to keep models current and optimized, inference is used many many times to serve millions of users. Two months after its launch, ChatGPT had 100 million active users. Instead of employing existing web searches that rely on smaller AI models, many people are eager to use AI for everything, but a single request in ChatGPT can consume 100 times more energy than one Google search, according to one tech expert.
Northeastern University and MIT researchers estimated that inference consumes more energy than training, but there is still debate over which mode is the greater energy consumer. What is certain, though, is that as OpenAI, Google, Microsoft, and the Chinese search company Baidu compete to create larger, more sophisticated models, and as more people use them, their carbon footprints will grow. This could potentially make decarbonizing our societies much more difficult.
“If you look at the history of computational advances, I think we’re in the ‘amazed by what we can do, this is great, let’s do it phase,’” said Clifford Stein, interim director of Columbia University’s Data Science Institute, and professor of computer science at the Fu Foundation School of Engineering and Applied Science. “But we should be coming to a phase where we’re aware of the energy usage and taking that into our calculations of whether we should or shouldn’t be doing it, or how big the model should be. We should be developing the tools to think about if it’s worth using these large language models given how much energy they’re consuming, and at least be aware of their energy and environmental costs.”
How can AI be made greener?
Many experts and researchers are thinking about the energy and environmental costs of artificial intelligence and trying to make it greener. Here are just some of the ways AI can be made more sustainable.
Transparency
You can’t solve a problem if you can’t measure it, so the first step towards making AI greener is to enable developers and companies to know how much electricity their computers are using and how that translates into carbon emissions. The measurements of AI carbon footprints also need to be standardized so that developers can compare the impacts of different systems and solutions. A group of researchers from Stanford, Facebook, and McGill University have developed a tracker to measure energy use and carbon emissions from training AI models. And Microsoft’s Emissions Impact Dashboard for Azure enables users to calculate their cloud’s carbon footprint.
Renewable energy use
According to Microsoft, all the major cloud providers have plans to run their cloud data centers on 100 percent carbon-free energy by 2030, and some already do. Microsoft is committed to running on 100 percent renewable energy by 2025, and has long-term contracts for green energy for many of its data centers, buildings, and campuses. Google’s data centers already get 100 percent of their energy from renewable sources.
Moving large jobs to data centers where the energy can be sourced from a clean energy grid also makes a big difference. For example, the training of AI startup Hugging Face’s large language model BLOOM with 176 billion parameters consumed 433 MWh of electricity, resulting in 25 metric tons of CO2 equivalent. It was trained on a French supercomputer run mainly on nuclear energy. Compare this to the training of GPT-3 with 175 billion parameters, which consumed 1287 MWh of electricity, and resulted in carbon emissions of 502 metric tons of carbon dioxide equivalent.
Better management of computers
Data centers can have thousands or hundreds of thousands of computers, but there are ways to make them more energy efficient. “Packing the work onto the computers in a more efficient manner will save electricity,” said Stein. “You may not need as many computers, and you can turn some off.”
He is also currently researching the implications of running computers at lower speeds, which is more energy efficient. In any data center, there are jobs that require an immediate response and those that don’t. For example, training takes a long time but usually doesn’t have a deadline. Computers could be run more slowly overnight, and it wouldn’t make a difference. For inference that’s done in real time, however, computers need to run quickly.
Another area of Stein’s research is the study of how accurate a solution needs to be when computing. “Sometimes we get solutions that are more accurate than the input data justifies,” he said. “By realizing that you only need to really be computing things approximately, you can often compute them much faster, and therefore in a more energy efficient manner.” For example, with some optimization problems, you are gradually moving towards some optimal solution. “Often if you look at how optimization happens, you get 99 percent of the way there pretty quickly, and that last one percent is what actually what takes half the time, or sometimes even 90 percent of the time” he said. The challenge is knowing how close you are to the solution so that you can stop earlier.
More efficient hardware
Chips that are designed especially for training large language models, such as tensor processing units developed by Google, are faster and more energy efficient than some GPUS.
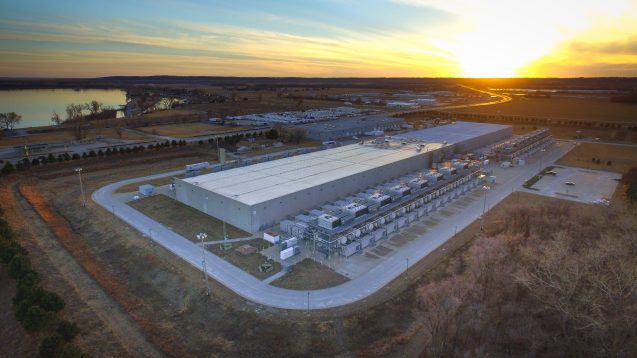
Google claims its data centers have cut their energy use significantly by using hardware that emits less heat and therefore needs less energy for cooling. Many other companies and researchers are also trying to develop more efficient hardware specifically for AI.
The right algorithms
Different algorithms have strengths and weaknesses, so finding the most efficient one depends on the task at hand, the amount and type of data used, and the computational resources available. “You have to look at the underlying problem [you’re trying to solve],” said Stein. “There are often many different ways you can compute it, and some are faster than others.”
People don’t always implement the most efficient algorithm. “Either because they don’t know them, or because it’s more work for the programmer, or they already have one implemented from five years ago,” he said. “So by implementing algorithms more efficiently, we could save electricity.” Some algorithms have also learned from experience to be more efficient.
The appropriate model
Large language models are not needed for every kind of task. Choosing to use a smaller AI model for simpler jobs is a way to save energy—more focused models instead of models that can do everything are more efficient. For instance, using large models might be worth the electricity they consume to try to find new antibiotics but not to write limericks.
Some researchers are trying to create language models using data sets that are 1/10,000 of the size in the large language models. Called the BabyLM Challenge, the idea is to get a language model to learn the nuances of language from scratch the way a human does, based on a dataset of the words children are exposed to. Each year, young children encounter between 2,000 to 7,000 words; for the BabyLM Challenge, the maximum number of words in the dataset is 100,000 words, which amounts to what a 13-year-old will have been exposed to. A smaller model takes less time and resources to train and thus consumes less energy.
Modifying the models
Fine tuning existing models instead of trying to develop even bigger new models would make AI more efficient and save energy.
Some AI models are “overparameterized.” Pruning the network to remove redundant parameters that do not affect a model’s performance could reduce computational costs and storage needed. The goal for AI developers is to find ways to reduce the number of parameters without sacrificing accuracy.
Knowledge distillation, transferring knowledge the model has learned from a massive network to a more compact one, is another way to reduce AI model size.
Virginia Tech and Amazon are studying federated learning, which brings the model training to the data instead of bringing data to a central server. In this system, parts of the model are trained on data stored on multiple devices in a variety of locations instead of in a centralized or cloud server. Federated learning reduces the amount of time training takes, and the amount of data that must be transferred and stored, all of which saves energy. Moreover, the data on the individual devices stays where it is, ensuring data security. After they are trained on the local devices, the updated models are sent back to a central server and aggregated into a new more comprehensive model.
New cooling methods
Because traditional cooling methods, such as air conditioning, cannot always keep data centers cool enough, Microsoft researchers are using a special fluid engineered to boil 90 degrees lower than water’s boiling point to cool computer processors. Servers are submerged into the fluid, which does not harm electronic equipment; the liquid removes heat from the hot chips and enables the servers to keep operating. Liquid immersion cooling is more energy efficient than air conditioners, reducing a server’s power consumption by 5 to 15 percent.
Microsoft is also experimenting with the use of underwater data centers that rely on the natural cooling of the ocean, and ocean currents and nearby wind turbines to generate renewable energy. Computers are placed in a cylindrical container and submerged underwater. On land, computer performance can be hampered by oxygen, moisture in the air, and temperature fluctuations. The underwater cylinder provides a stable environment without oxygen. Researchers say that underwater computers have one-eighth the failure rate as those on land.
Eventually, data centers may move from the cloud into space. A startup called Lonestar has raised five million dollars to build small data centers on the moon by the end of 2023. Lunar data centers could take advantage of abundant solar energy and would be less susceptible to natural disasters and sabotage.
Thales Alenia Space is leading a study on the feasibility of building data centers in space which would run on solar energy. The study is attempting to determine if the launch and production of space data centers would result in fewer carbon emissions than those on land.
Government support for sustainable AI
To speed the development of more sustainable AI, governments need to establish regulations for the transparency of its carbon emissions and sustainability. Tax incentives are also needed to encourage cloud providers to build data centers where renewable energy is available, and to incentivize the expansion of clean energy grids.
“If we’re smart about AI, it should be beneficial [to the planet] in the long run,” said Stein. “We certainly have the ability to be smart about it, to use it to get all kinds of energy savings. I think AI will be good for the environment, but to achieve that requires us to be thoughtful and have good leadership.”